How to take your AI pilot to production by making the right Large Language Model choices
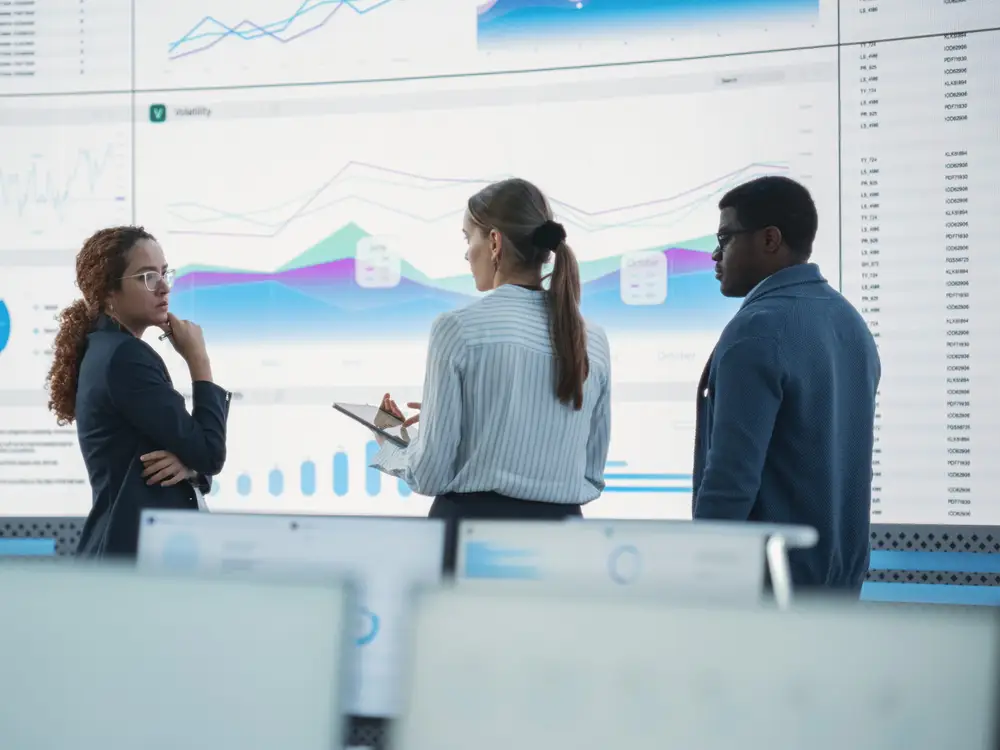
The right AI strategy includes the right LLM choice
By Kate Woolley, General Manager, IBM Ecosystem
By now, we have seen the numbers: 75% of organizations are piloting generative AI in five or more business functions, and nearly 50% of CEOs expect to use GenAI to drive growth and expansion by 2026. As companies increasingly scale GenAI pilots, the blueprint for transforming operations across use cases such as customer service, workforce productivity, and code modernization is becoming more apparent.
One of the most common denominators among successful AI implementations is that enterprises aren’t doing it alone. They’re enlisting partners with the right mix of industry and technology expertise to build and execute a responsible AI strategy that is right for their business, including the important selection of Large Language Models (LLM) that are best suited to their needs.
Being well informed when choosing the right LLM, or multiple LLMs, is critical to the success of not just one targeted project but also the long-term viability of a company’s AI strategy. A misstep could have ramifications in terms of risk, cost, compute resources, and flexibility.
While there are many considerations to selecting LLMs, we’ve found the following four to be the most foundational for a winning, sustainable approach to enterprise AI.
1.A catch-all AI model isn’t necessarily the best model for your business
Many LLMs are built for general-purpose use and take a one-size-fits-all approach. This can be costly and limiting for an enterprise that requires specialized features and a more nuanced understanding of its data. Enterprises should be able to choose domain-specific models, customize them for a particular use case, company, and/or industry, and train them on their data. That doesn’t always mean a full retuning of the model, but putting a layer of customization on top. Additionally, bigger isn’t always better — smaller and more targeted models like the IBM Granite family of models can perform on par with larger general-purpose models, while offering the flexibility to adapt them to new scenarios and use cases.
2.Models that require fewer compute resources can help you scale GenAI in a cost-effective way
In the enterprise, it’s not enough for models to work — they must do so efficiently and at a cost that makes sense for scaling. For example, some LLMs are cost-prohibitive for inferencing due to compute needs, GPU requirements, or even carbon footprint. The ultimate formula is a high-performance model with lower inferencing costs and fewer computing resources, enabling organizations to scale generative AI more cost-effectively and sustainably. Models that are easier to tune and integrate with applications also impact efficiency. LLMs that can be deployed either in a hybrid environment or in a company’s data center are additional considerations.
3.Ensure trust and transparency by avoiding ‘black box’ LLMs
Many LLMs often fail to provide transparency into the data used to train the model or do not provide the ability to tune the model with enterprise data. Every organization should be in control of its AI, infusing accountability and oversight. They should also own the IP when a model is refined. The ability to inspect the model’s code, understand its workings, and trust its outputs is crucial to ensure trustworthy AI that can be audited for biases and other ethical concerns. It makes a real difference for chief information officers, chief legal officers, and compliance officers to know that the model used is infused with trusted, relevant data.
4.Open-sourced LLMs leverage an ecosystem of shared insights
An open AI ecosystem empowers the collective to explore, test, study, and deploy AI more transparently – cultivating a significantly broader and more diverse pool of AI talent that can contribute to rapid model innovation. An open approach helps to inform the development of standards and best practices within the AI community, promoting interoperability between different systems and technologies. Additionally, new tuning techniques such as InstructLab mean source code can be improved by the open community — then rapidly tuned for specific needs. This approach also aligns with the principles of ethical AI by providing a platform for accountability and oversight, IP ownership, sovereignty, and indemnification with the goal of reducing risk.
Applying these considerations early in your AI journey, with the guidance and support of trusted partners, will help ensure that the right decision-making happens upfront, including selecting the right models that can be scaled to better fit the cost and performance requirements of your specific business needs. With this foundation in place, you will be empowered to accelerate AI pilots out of the lab and into the hands of business users, where they can more quickly deliver results.