Sovereign AI explained: An AI cloud CEO unpacks what’s behind the trend
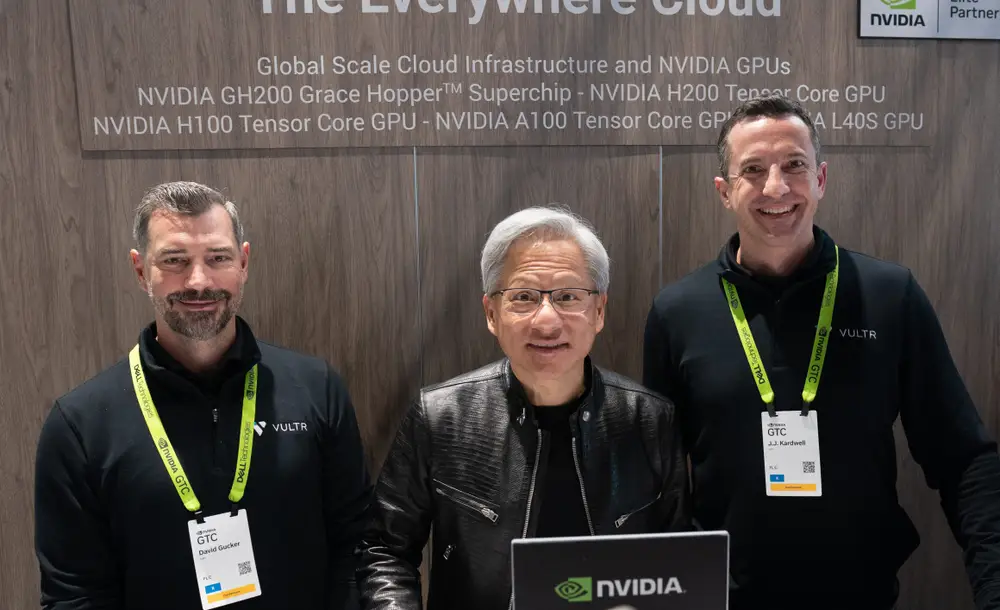
Vultr executives CEO J.J. Kardwell (right) and COO David Gucker were early customers of Nvidia.
Most of our online work happens in the cloud these days, though we likely don’t know where the computing is actually happening. The location of the data center that houses our spreadsheets and shopping lists doesn’t matter much to most of us until it does.
In the age of AI, where computations are becoming more complex by the day, some nations are looking to make sure some of that power is within their borders. It’s good business, but it’s also a matter of pride, practicality, and national security.
Vultr is a cloud service provider and data center operator founded in 2014. The company started buying Nvidia GPUs in 2021, getting to the AI cloud computing business earlier than most. It operates data centers globally, with both traditional computing and AI servers for public cloud clients, along with companies and countries that want their own private clouds. CEO J.J. Kardwell describes the company as the largest privately-held cloud computing platform in the world.
A large global footprint, with more than 30 data centers on six continents, helps with computing speed, especially for AI inference. It also makes the Vultr boss well-positioned to explain a phrase frequently on the lips of AI luminaries like Nvidia CEO Jensen Huang: sovereign AI.
B-17: What is a sovereign cloud?
Sovereign cloud is the delivery of cloud infrastructure as a service, typically inside a country. It guarantees data is stored locally, which ensures it’s used strictly for the intended purposes and not transferred outside of national borders without explicit authorization. That’s the essence of it.
The higher levels of sovereignty involve no communication, no information whatsoever, even at a control plane level, passing outside of a country. Every single interaction, even with a platform, happens in-country. This helps ensure data sovereignty, data residency, data governance, and compliance with local privacy laws.
B-17: If most companies simply rent computing capacity from a cloud provider, why would they care where the data centers are?
In some cases, it’s actual compliance with the law. For example, let’s say it was a patient’s medical records, personally identifiable information, or other sensitive data. They may have a compliance obligation regarding where they can store data.
The second is that the lowest level of risk is if the chain of custody can be documented from end to end. So keeping data in-country and in a sovereign cloud enables the highest levels of accountability and avoids transferring data across international networks.
B-17: How does a sovereign cloud work?
If you want true technological independence, you can deliver it with essentially an air gap deployment and a dedicated control plane where the customer is completely untethered from any other data centers or infrastructure.
AI doesn’t necessarily require interactions with data from many sources. Many training jobs are specific data sets that can be loaded onto a network in a sovereign deployment. And everything can quite easily be executed in-country.
I think many countries realize that some of the most important applications of AI are around matters of national security, critical industries, and citizen data, where their local laws around consumer data and privacy may be very, very different from those in other countries. So it’s quite important to them to be able to ensure for their citizens that anything that’s being done, with AI is being done within the confines of the country and in compliance with their own laws.
B-17: What do people mean when they use the term “sovereign AI”?
They are focused on capacity in a specific country, and typically they’re talking about capacity sponsored by the government, whether it’s at a state or federal level within that country.
One of the challenges, or inequities, is the very uneven distribution of AI infrastructure around the world.
Obviously, people are well aware of the transformative impact that AI can have across so many industries, but the infrastructure itself to perform that work, train models, and support inference is very unevenly distributed globally.
Many countries are realizing that if they don’t take an active role at the government level in helping sponsor, encourage, and incentivize the deployment of infrastructure locally, their businesses and populations will have less access to that technology.
By definition, large language models are specific to a language. And the biggest portions of early innovation were done in US English. Only recently we’re seeing the globalization of many of these models. You can’t train on a US body of data, for example, for legal documentation then do simple translation into other languages.
Obviously, you’d miss local conventions of law and language, and therefore, translations are not enough. You’d actually need to do training on local models, on local bodies of data. Every major language economy in the world wants to benefit from AI, yet the resource is not available proportionately to where the population or even the GDP of the world is, right now.
B-17: AI computing is extremely energy intensive, and concerns about the US grid abound. Can you speak to how those dynamics are changing where data centers might be built?
Given the significant growing power consumption of AI infrastructure and the importance of its efficient delivery, we will increasingly see capacity building in global markets that have a comparative advantage in supporting lower-cost data center capacity.
We have a strategic partnership with Singtel in Singapore, and if you look at the way that they’re building across the region, they’re bringing up massive capacity in markets like Malaysia and others where we’ll be able to very efficiently serve the region and much of the world where you see more efficient power and operating costs.
The domestic military or Department of Defense equivalent in any country will want those deployments to be domestic, regardless of any cost.
But for many enterprises, so long as the execution is done at world class compliance, privacy, and trust commitments, you will see more efficient distribution of those workloads that can be executed in other places in the world.