Will the world’s fastest supercomputer please stand up?
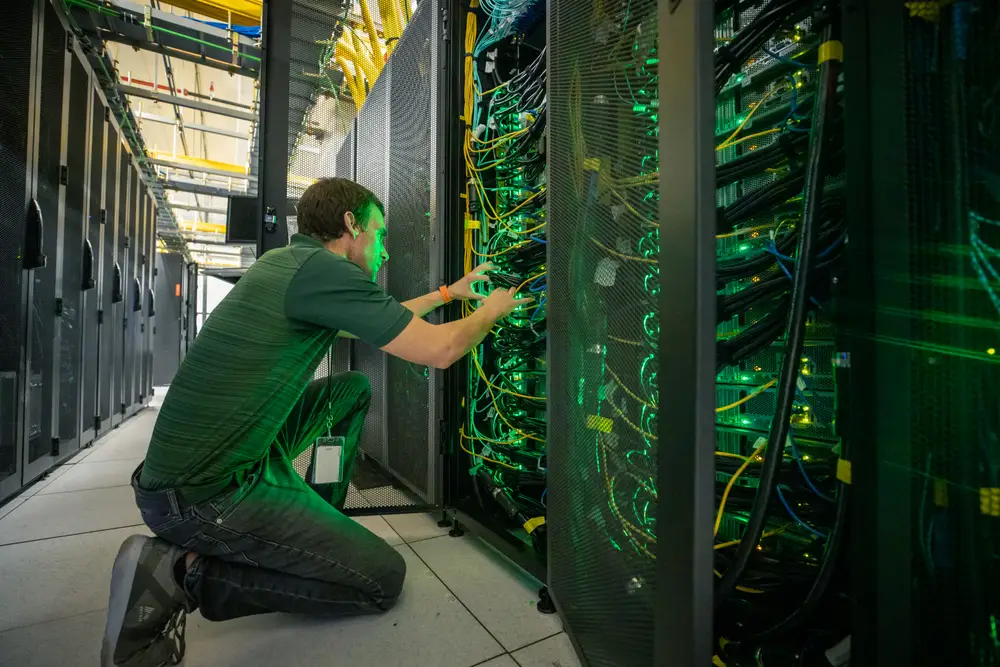
TRITON Supercomputer at the University of Miami
In high school, as in tech, superlatives are important. Or maybe they just feel important in the moment. With the breakneck pace of the AI computing infrastructure buildout, it’s becoming increasingly difficult to keep track of who has the biggest, fastest, or most powerful supercomputer — especially when multiple companies claim the title at once.
“We delivered the world’s largest and fastest AI supercomputer, scaling up to 65,000 Nvidia H200 GPUs,” Oracle CEO Safra Catz and Chairman, CTO, echoed by Founder Larry Ellison on the company’s Monday earnings call.
In late October, Nvidia proclaimed xAI’s Colossus as the “World’s Largest AI Supercomputer,” after Elon Musk’s firm reportedly built a computing cluster with 100,000 Nvidia graphics processing units in a matter of weeks. The plan is to expand to 1 million GPUs next, according to the Greater Memphis Chamber of Commerce (where the supercomputer is located.)
The good ole days of supercomputing are gone
It used to be simpler. “Supercomputers” were most commonly found in research settings. Naturally, there’s an official list ranking supercomputers. Until recently the world’s most powerful supercomputer was named El Capitan. Housed at the Lawrence Livermore National Laboratory in California 11 million CPUs and GPUs from Nvidia-rival AMD add up to 1.742 Exaflops of computing capacity. (One exaflop is equal to one quintillion, or a billion billions, operations per second.)
“The biggest computers don’t get put on the list,” Dylan Patel, chief analyst at Semianalysis, told B-17. “Your competitor shouldn’t know exactly what you have,” he continued. The 65,000-GPU supercluster Oracle executives were praising can reach up to 65 exaflops, according to the company.
It’s safe to assume, Patel said, that Nvidia’s largest customers, Meta, Microsoft, and xAI also have the largest, most powerful clusters. Nvidia CFO Colette Cress said 200 fresh exaflops of Nvidia computing would be online by the end of this year — across nine different supercomputers — on Nvidia’s May earnings call.
Going forward, it’s going to be harder to determine whose clusters are the biggest at any given moment — and even harder to tell whose are the most powerful — no matter how much CEOs may brag.
It’s not the size of the cluster — it’s how you use it
On Monday’s call, Ellison was asked, if the size of these gigantic clusters is actually generating better model performance.
He said larger clusters and faster GPUs are elements that speed up model training. Another is networking it all together. “So the GPU clusters aren’t sitting there waiting for the data,” Ellison said Monday.
Thus, the number of GPUs in a cluster isn’t the only factor in the computing power calculation. Networking and programming are important too. “Exaflops” are a result of the whole package so unless companies provide them, experts can only estimate.
What’s certain is that more advanced models — the kind that consider their own thinking and check their work before answering queries — require more compute than their relatives of earlier generations. So training increasingly impressive models may indeed require an arms race of sorts.
But an enormous AI arsenal doesn’t automatically lead to better or more useful tools.
Sri Ambati, CEO of open-source AI platform H2O.ai, said cloud providers may want to flex their cluster size for sales reasons, but given some (albeit slow) diversification of AI hardware and the rise of smaller, more efficient models, cluster size isn’t the end all be all.
Power efficiency too, is a hugely important indicator for AI computing since energy is an enormous operational expense in AI. But it gets lost in the measuring contest.
Nvidia declined to comment. Oracle did not respond to a request for comment in time for publication.